The Evaluation of Models, Inferences, and Experimental Designs for ACT Exam is a critical aspect of the ACT Science section. This topic encompasses the ability to assess scientific models, draw inferences from data, and evaluate the design and methodology of experiments. Understanding how to critically evaluate these elements is essential for interpreting scientific information accurately and making informed decisions based on empirical evidence.
Learning Objectives
By the end of this topic, you will be able to critically evaluate scientific models, assess the validity of inferences drawn from data, and analyze the strengths and weaknesses of experimental designs, thus enhancing their ability to understand and interpret scientific information accurately and effectively.
Understanding Scientific Models
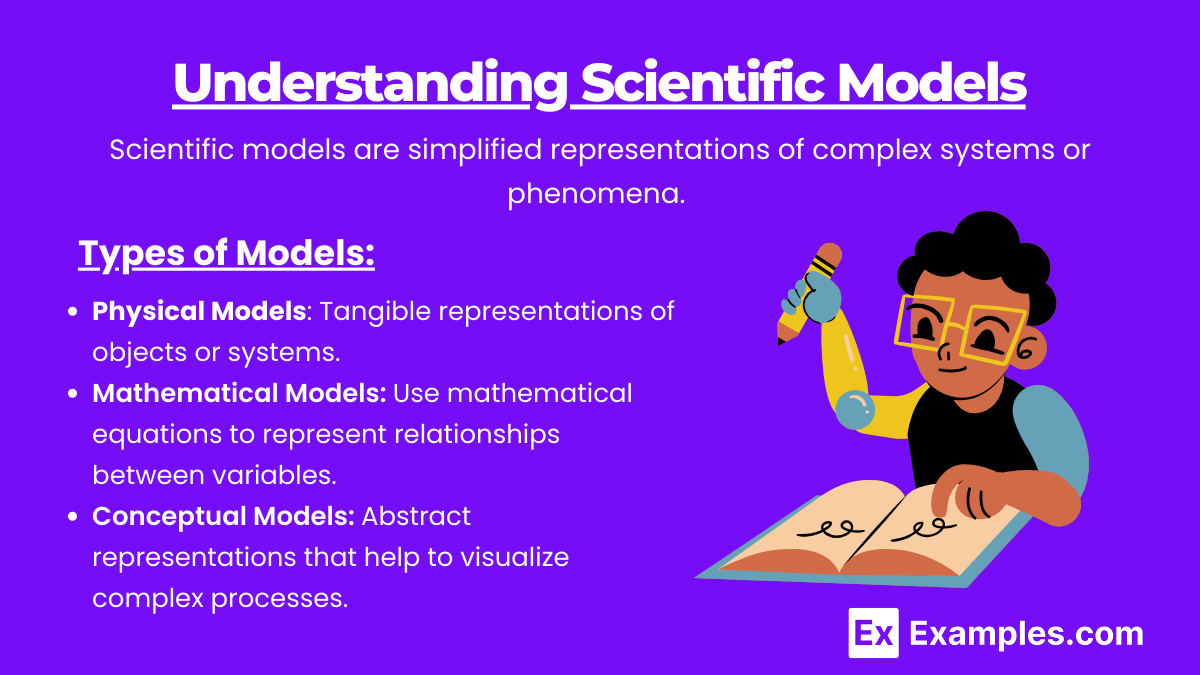
Scientific models are simplified representations of complex systems or phenomena. They help scientists to predict and explain behaviors and outcomes in the natural world. Models can be physical, mathematical, or conceptual and are used across various scientific disciplines.
Types of Models
- Physical Models: Tangible representations of objects or systems (e.g., a globe representing the Earth).
- Mathematical Models: Use mathematical equations to represent relationships between variables (e.g., the equation of a line in physics).
- Conceptual Models: Abstract representations that help to visualize complex processes (e.g., the water cycle diagram).
Evaluation of Models
Evaluating a model involves assessing its accuracy, consistency with empirical data, and predictive power. Questions to consider include:
- How well does the model fit the observed data?
- Does the model make accurate predictions?
- Is the model consistent with other established scientific theories?
Assessing Inferences
Inferences are conclusions drawn from data and observations. In scientific contexts, inferences are critical as they lead to hypotheses, theories, and further experimentation.
Types of Inferences
- Deductive Inference: Conclusions drawn from general principles or premises (e.g., All humans are mortal; Socrates is a human; therefore, Socrates is mortal).
- Inductive Inference: Generalizations made from specific observations (e.g., observing that the sun rises in the east every day and inferring that it will rise in the east tomorrow).
Evaluating Inferences
To evaluate inferences, consider the following:
- Are the premises or observations accurate and reliable?
- Is the reasoning logically sound?
- Are there alternative explanations that have not been considered?
Analyzing Experimental Designs
Experimental design refers to how researchers set up an experiment to test a hypothesis. Key components include the independent variable, dependent variable, control group, and experimental group.
Types of Experimental Designs
- Controlled Experiments: Experiments where all variables are kept constant except for the independent variable.
- Field Experiments: Conducted in a natural setting rather than a laboratory.
- Quasi-Experiments: Lack random assignment of participants to groups but still involve manipulation of an independent variable.
Evaluating Experimental Designs
Key aspects to consider when evaluating experimental designs include:
- Validity: Does the experiment measure what it claims to measure? This includes internal validity (accuracy of the results) and external validity (generalizability of the results).
- Reliability: Can the results be replicated under the same conditions?
- Bias and Error: Are there any biases or errors that could affect the results?
Integration of Evaluation of Models, Inferences, and Experimental
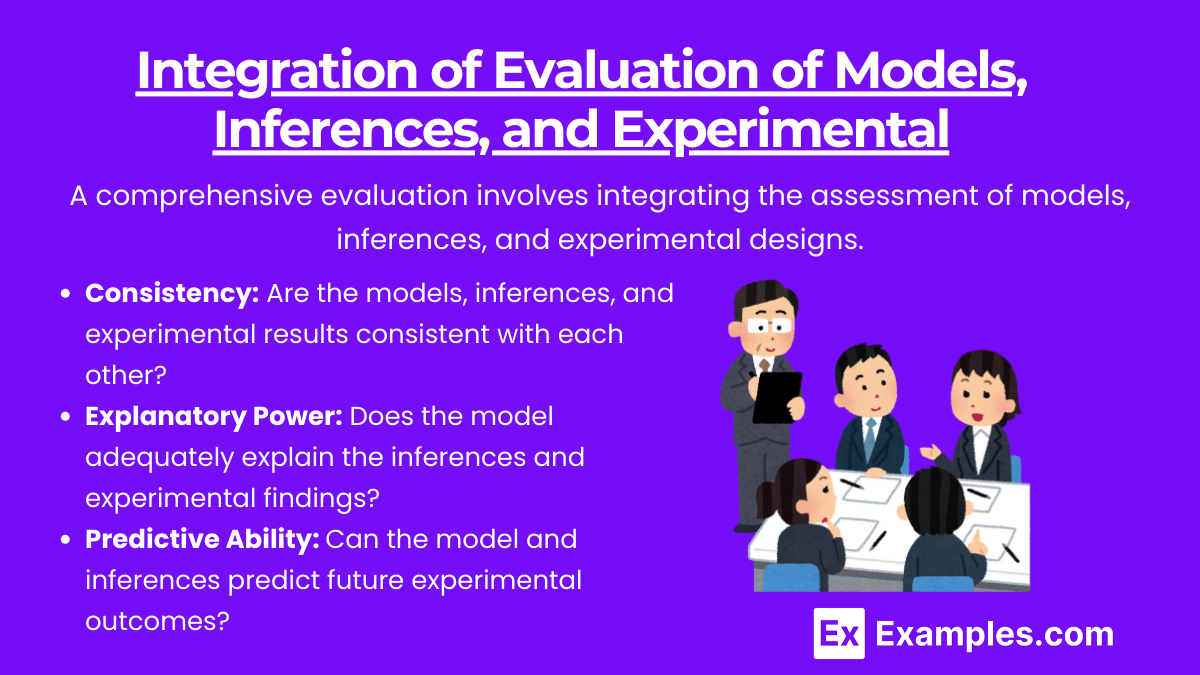
A comprehensive evaluation involves integrating the assessment of models, inferences, and experimental designs. This holistic approach ensures a thorough understanding of the scientific inquiry process.
- Consistency: Are the models, inferences, and experimental results consistent with each other?
- Explanatory Power: Does the model adequately explain the inferences and experimental findings?
- Predictive Ability: Can the model and inferences predict future experimental outcomes?
Examples
Example 1: Evaluating a Biological Model
Scenario: Scientists develop a model predicting the growth rate of bacteria in different temperatures.
Evaluation:
- Accuracy: Compare the predicted growth rates to observed data from laboratory experiments.
- Consistency with Empirical Data: Validate the model with multiple sets of experimental data under various temperature conditions.
- Predictive Power: Assess if the model can predict growth rates in temperatures not yet tested.
- Consistency with Scientific Theories: Ensure the model aligns with established principles of microbiology.
- Generalizability: Check if the model applies to different types of bacteria and other similar organisms.
Example 2: Assessing an Environmental Inference
Scenario: Researchers infer that increased CO2 levels lead to higher plant growth based on observations from a controlled greenhouse experiment.
Evaluation:
- Accuracy: Determine if the data supporting the inference is reliable and reproducible.
- Consistency with Empirical Data: Cross-check the inference with data from different experiments and natural environments.
- Predictive Power: Evaluate if this inference can predict plant growth in other controlled and natural environments.
- Consistency with Scientific Theories: Align the inference with the known effects of CO2 on photosynthesis and plant physiology.
- Generalizability: Test the inference with various plant species and under different environmental conditions.
Example 3: Analyzing a Physics Experimental Design
Scenario: A physics experiment tests the relationship between the angle of incidence and the angle of refraction of light through a prism.
Evaluation:
- Accuracy: Ensure the measurements of angles are precise and accurate.
- Consistency with Empirical Data: Validate results by repeating the experiment and comparing with known data.
- Predictive Power: Assess if the experimental design can predict angles of refraction for different materials.
- Consistency with Scientific Theories: Ensure the experiment aligns with Snell’s Law and principles of optics.
- Robustness: Test the experiment under different conditions to ensure consistent results.
Example 4: Evaluating a Chemical Model
Scenario: A chemical model predicts the reaction rates of an enzyme-catalyzed reaction at different pH levels.
Evaluation:
- Accuracy: Compare the model’s predicted reaction rates with actual experimental data.
- Consistency with Empirical Data: Validate the model using data from multiple experiments conducted at different pH levels.
- Predictive Power: Determine if the model can accurately predict reaction rates outside the tested pH range.
- Consistency with Scientific Theories: Ensure the model is consistent with known principles of enzyme kinetics and biochemistry.
- Generalizability: Check if the model applies to different enzymes and substrates.
Example 5: Assessing a Geological Inference
Scenario: Geologists infer the age of a rock layer based on the presence of certain fossils.
Evaluation:
- Accuracy: Verify the fossil identification and dating techniques used.
- Consistency with Empirical Data: Compare the inference with other dating methods and fossil records.
- Predictive Power: Assess if the inference can predict the age of rock layers in different geographic locations.
- Consistency with Scientific Theories: Ensure alignment with established geological time scales and principles of stratigraphy.
- Generalizability: Test the inference with different types of rock layers and fossil assemblages.
Practice Questions
Question 1
Question: A research team developed a model to predict the growth of a certain plant species under different light conditions. They observed that the model accurately predicts growth under low and medium light but overestimates growth under high light conditions.
Which of the following steps should the researchers take to improve their model?
A. Increase the sample size of plants grown under low and medium light conditions.
B. Validate the model with additional data from plants grown under high light conditions.
C. Simplify the model to reduce the number of variables.
D. Disregard the data from high light conditions as an outlier.
Answer: B
Explanation: To improve the model’s accuracy under high light conditions, the researchers should validate it with additional data from plants grown under those conditions. This step will help identify and correct the discrepancies in the model’s predictions.
Question 2
Question: An experiment was conducted to test the hypothesis that increasing the concentration of a reactant will increase the rate of a chemical reaction. The results showed a positive correlation between reactant concentration and reaction rate, but with considerable variation in the data.
What should the researchers consider to evaluate the reliability of their inference?
A. The consistency of the results with existing scientific theories.
B. The number of trials conducted at each concentration level.
C. The alignment of the results with their initial hypothesis.
D. The presence of any potential biases or errors in the experimental design.
Answer: D
Explanation: To evaluate the reliability of their inference, researchers should consider potential biases or errors in the experimental design that could account for the variation in the data. Ensuring the experiment was conducted without biases or errors will help determine if the observed correlation is truly reliable.
Question 3
Question: A group of students is evaluating a geological model that predicts the age of rock layers based on the types of fossils found within them. They find that the model accurately dates rock layers in one region but gives inconsistent results in another region with different fossil types.
Which of the following should the students do to evaluate the model’s generalizability?
A. Use the model to predict the age of rock layers in a third, untested region.
B. Compare the model’s predictions with another dating method in the inconsistent region.
C. Adjust the model to only apply to the region where it gave accurate results.
D. Conduct more tests in the region where the model gave inconsistent results.
Answer: B
Explanation: To evaluate the model’s generalizability, the students should compare its predictions with another dating method in the region where it gave inconsistent results. This comparison will help determine whether the model can be applied broadly or if it needs adjustments for different regions.