Chi Square Test
The chi-square test, a cornerstone of statistical analysis, is utilized to examine the independence of two categorical variables, offering a method to assess observed versus expected frequencies in categorical data. This test extends beyond basic algebra and rational numbers, involving computations with square and square roots, which are integral in determining the chi-square statistic. Unlike dealing with integers or continuous rational and irrational numbers directly, this test quantifies how much observed counts deviate from expected counts in categorical data, rooted in the realm of probability and discrete mathematics. Additionally, while it diverges from the least squares method used for continuous data regression, both share a common goal of minimizing deviation to optimize fit between observed and expected models. In statistics, understanding and applying the chi-square test provides crucial insights into data relationships, crucial for robust analytical conclusions in research and real-world applications.
What is Chi Square Test?
Chi-Square Distribution
The chi-square distribution is a fundamental probability distribution in statistics, widely used in hypothesis testing and confidence interval estimation for variance. It arises primarily when summing the squares of independent, standard normal variables, and is characterized by its degrees of freedom, which influence its shape. As the degrees of freedom increase, the distribution becomes more symmetric and approaches a normal distribution. This distribution is crucial in constructing the chi-square test for independence and goodness-of-fit tests, helping to determine whether observed frequencies significantly deviate from expected frequencies under a given hypothesis. It is also integral to the analysis of variance (ANOVA) and other statistical procedures that assess the variability among group means.
Finding P-Value
Step 1: Understand the P-Value
The p-value represents the probability of observing a test statistic as extreme as, or more extreme than, the value calculated from the sample data, under the null hypothesis. A low p-value (typically less than 0.05) suggests that the observed data is inconsistent with the null hypothesis, leading to its rejection.
Step 2: Calculate the Test Statistic
Depending on the statistical test being used (like t-test, chi-square test, ANOVA, etc.), first calculate the appropriate test statistic based on your data. This involves different formulas depending on the test and the data structure.
Step 3: Determine the Distribution
Identify the distribution that the test statistic follows under the null hypothesis. For example, the test statistic in a chi-square test follows a chi-square distribution, while a t-test statistic follows a t-distribution.
Step 4: Find the P-Value
Use the distribution identified in Step 3 to find the probability of obtaining a test statistic as extreme as the one you calculated. This can be done using statistical software, tables, or online calculators. You will compare your test statistic to the critical values from the distribution, calculating the area under the curve that lies beyond the test statistic.
Step 5: Interpret the P-Value
- If the p-value is less than the chosen significance level (usually 0.05), reject the null hypothesis, suggesting that the effect observed in the data is statistically significant.
- If the p-value is greater than the significance level, you do not have enough evidence to reject the null hypothesis, and it is assumed that any observed differences could be due to chance.
Practical Example
For a simpler illustration, suppose you’re conducting a two-tailed t-test with a t-statistic of 2.3, and you’re using a significance level of 0.05. You would:
- Identify that the t-statistic follows a t-distribution with degrees of freedom dependent on your sample size.
- Using a t-distribution table or software, find the probability that a t-value is at least as extreme as Β±2.3.
- Sum the probabilities of obtaining a t-value of 2.3 or higher and -2.3 or lower. This sum is your p-value.
Properties of Chi-Square
1. Non-Negativity
- The chi-square statistic is always non-negative. This property arises because it is computed as the sum of the squares of standardized differences between observed and expected frequencies.
2. Degrees of Freedom
- The shape and scale of the chi-square distribution are primarily determined by its degrees of freedom, which in turn depend on the number of categories or variables involved in the analysis. The degrees of freedom for a chi-square test are generally calculated as (πβ1)(πβ1) for an πΓπ contingency table.
3. Distribution Shape
- The chi-square distribution is skewed to the right, especially with fewer degrees of freedom. As the degrees of freedom increase, the distribution becomes more symmetric and starts to resemble a normal distribution.
4. Additivity
- The chi-square distributions are additive. This means that if two independent chi-square variables are added together, their sum also follows a chi-square distribution, with degrees of freedom equal to the sum of their individual degrees of freedom.
5. Dependency on Sample Size
- The chi-square statistic is sensitive to sample size. Larger sample sizes tend to give more reliable estimates of the chi-square statistic, reducing the influence of sampling variability. This property emphasizes the need for adequate sample sizes in experiments intending to use chi-square tests for valid inference.
Chi-Square Formula
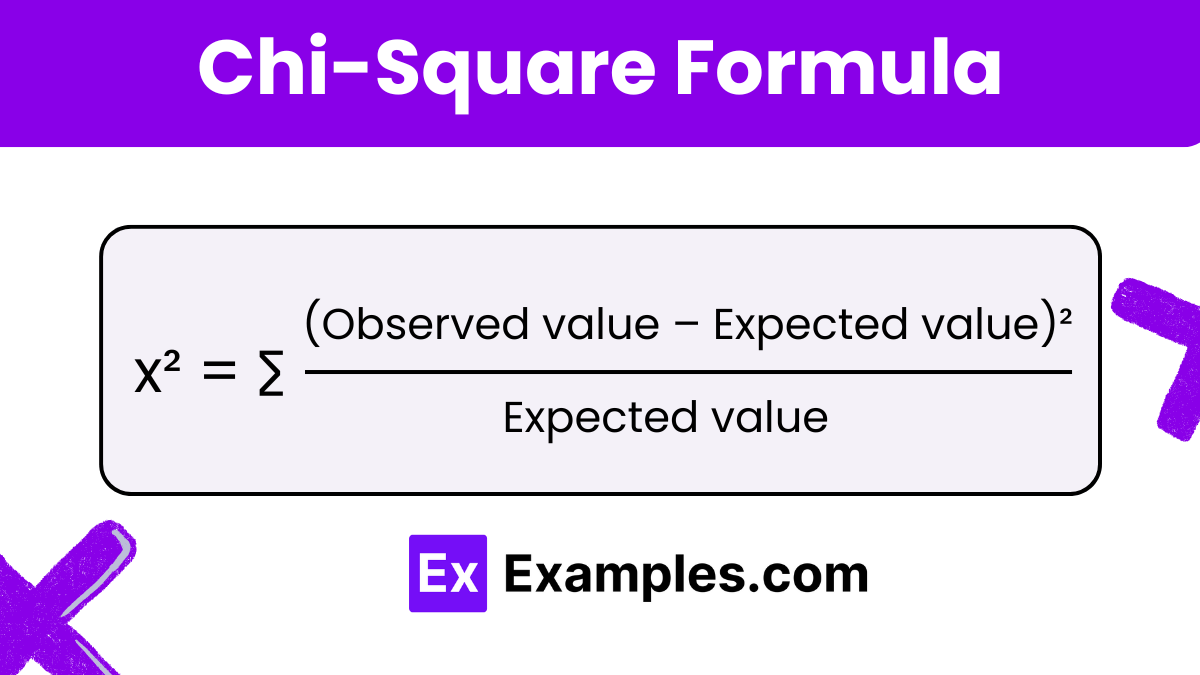
Components of the Formula:
- ΟΒ² is the chi-square statistic.
- πα΅’β represents the observed frequency for each category.
- πΈα΅’β represents the expected frequency for each category, based on the hypothesis being tested.
- The summation (β) is taken over all categories involved in the test.
Chi-Square Test of Independence
Purpose
The Chi-Square Test of Independence assesses whether two categorical variables are independent, meaning whether the distribution of one variable differs depending on the value of the other variable.
Assumptions
Before conducting the test, certain assumptions must be met:
- Sample Size: All expected frequencies should be at least 1, and no more than 20% of expected frequencies are less than 5.
- Independence: Observations must be independent of each other, typically achieved by random sampling.
- Data Level: Both variables should be categorical (nominal or ordinal).
Example of Categorical Data
Pet Ownership | Prefers Organic Pet Food | Prefers Non-Organic Pet Food | Total |
---|---|---|---|
Owns a Pet | 120 | 80 | 200 |
Does Not Own a Pet | 60 | 140 | 200 |
Total | 180 | 220 | 400 |
Breakdown of the Table
- Rows: Represent different categories of pet ownership (Owns a Pet, Does Not Own a Pet).
- Columns: Represent preferences for types of pet food (Organic, Non-Organic).
- Cells: Show the frequency of respondents in each combination of categories (e.g., 120 people own a pet and prefer organic pet food).
Table
Below is the representation of a chi-square distribution table with three probability levels (commonly used significance levels: 0.05, 0.01, and 0.001) for degrees of freedom up to 50. The degrees of freedom (DF) for a chi-square test in a contingency table are calculated as (r-1)(c-1), where r is the number of rows and c is the number of columns. This table is vital for determining critical values when testing hypotheses involving categorical data.
Degrees of Freedom (DF) | ΟΒ² at p=0.05 | ΟΒ² at p=0.01 | ΟΒ² at p=0.001 |
---|---|---|---|
1 | 3.84 | 6.63 | 10.83 |
2 | 5.99 | 9.21 | 13.82 |
3 | 7.81 | 11.34 | 16.27 |
4 | 9.49 | 13.28 | 18.47 |
5 | 11.07 | 15.09 | 20.52 |
6 | 12.59 | 16.81 | 22.46 |
7 | 14.07 | 18.48 | 24.32 |
8 | 15.51 | 20.09 | 26.12 |
9 | 16.92 | 21.67 | 27.88 |
10 | 18.31 | 23.21 | 29.59 |
11 | 19.68 | 24.72 | 31.26 |
12 | 21.03 | 26.22 | 32.91 |
13 | 22.36 | 27.69 | 34.53 |
14 | 23.68 | 29.14 | 36.12 |
15 | 24.99 | 30.58 | 37.70 |
16 | 26.30 | 32.00 | 39.25 |
17 | 27.59 | 33.41 | 40.79 |
18 | 28.87 | 34.81 | 42.31 |
19 | 30.14 | 36.19 | 43.82 |
20 | 31.41 | 37.57 | 45.32 |
21 | 32.67 | 38.93 | 46.80 |
22 | 33.92 | 40.29 | 48.27 |
23 | 35.17 | 41.64 | 49.73 |
24 | 36.42 | 42.98 | 51.18 |
25 | 37.65 | 44.31 | 52.62 |
26 | 38.89 | 45.64 | 54.05 |
27 | 40.11 | 46.96 | 55.48 |
28 | 41.34 | 48.28 | 56.89 |
29 | 42.56 | 49.59 | 58.30 |
30 | 43.77 | 50.89 | 59.70 |
31 | 44.99 | 52.19 | 61.09 |
32 | 46.19 | 53.49 | 62.48 |
33 | 47.40 | 54.78 | 63.87 |
34 | 48.60 | 56.06 | 65.25 |
35 | 49.80 | 57.34 | 66.62 |
36 | 51.00 | 58.62 | 67.99 |
37 | 52.19 | 59.89 | 69.36 |
38 | 53.38 | 61.16 | 70.72 |
39 | 54.57 | 62.43 | 72.07 |
40 | 55.76 | 63.69 | 73.42 |
41 | 56.94 | 64.95 | 74.77 |
42 | 58.12 | 66.21 | 76.11 |
43 | 59.30 | 67.46 | 77.45 |
44 | 60.48 | 68.71 | 78.79 |
45 | 61.66 | 69.96 | 80.12 |
46 | 62.83 | 71.20 | 81.45 |
47 | 64.00 | 72.44 | 82.78 |
48 | 65.17 | 73.68 | 84.10 |
49 | 66.34 | 74.92 | 85.42 |
50 | 67.50 | 76.15 | 86.74 |
This table provides critical values for various degrees of freedom and significance levels, which can be used to determine the likelihood of observing a chi-square statistic at least as extreme as the test statistic calculated from your data, under the assumption that the null hypothesis is true.
Example of Chi-Square Test for Independence
The Chi-square test for independence is a statistical test commonly used to determine if there is a significant relationship between two categorical variables in a population. Let’s go through a detailed example to understand how to apply this test.
Context
Imagine a researcher wants to investigate whether gender (male or female) affects the choice of a major (science or humanities) among university students.
Data Collection
The researcher surveys a sample of 300 students and compiles the data into the following contingency table:
Major | Male | Female | Total |
---|---|---|---|
Science | 70 | 80 | 150 |
Humanities | 60 | 90 | 150 |
Total | 130 | 170 | 300 |
Hypotheses
- Null Hypothesis (Hβ): There is no relationship between gender and choice of major.
- Alternative Hypothesis (Hβ): There is a relationship between gender and choice of major.
1. Calculate Expected Counts:
- Under the null hypothesis, if there’s no relationship between gender and major, the expected count for each cell of the table is calculated by the formula:
Eα΅’β±Ό β= (Row TotalΓColumn Total)β/Total Observations
For the ‘Male & Science’ cell:
πΈββββ, βκα΅’ββκβ = (150Γ130)/300 = 65
Repeat this for each cell.
Compute Chi-Square Statistic
The chi-square statistic is calculated using:
ΟΒ² = β(OβE)Β²β/E
Where π is the observed frequency, and πΈ is the expected frequency. For each cell:
ΟΒ² = 65(70β65)Β²β+85(80β85)Β²β+65(60β65)Β²β+85(90β85) β = 1.615
Determine Significance
With 1 degree of freedom (df = (rows – 1)/ times (columns – 1)), check the critical value from the chi-square distribution table at the desired significance level (e.g., 0.05). If πΒ² calculated is greater than the critical value from the table, reject the null hypothesis.
FAQs
What does the Chi-Square value indicate?
The Chi-Square value indicates how much the observed frequencies deviate from the expected frequencies under the null hypothesis of independence. A higher Chi-Square value suggests a greater deviation, which may lead to the rejection of the null hypothesis if the value exceeds the critical value from the Chi-Square distribution table for the given degrees of freedom and significance level.
How do you interpret the results of a Chi-Square Test?
To interpret the results of a Chi-Square Test, compare the calculated Chi-Square statistic to the critical value from the Chi-Square distribution table at your chosen significance level (commonly 0.05 or 0.01). If the calculated value is greater than the critical value, reject the null hypothesis, suggesting a significant association between the variables. If it is less, fail to reject the null hypothesis, indicating no significant association.
What are the limitations of the Chi-Square Test?
The Chi-Square Test assumes that the data are from a random sample, observations are independent, and expected frequencies are sufficiently large, typically at least 5 in each cell of the table. When these conditions are not met, the test results may not be valid. Additionally, the test does not provide information about the direction or strength of the association, only its existence.